本レポートでは、AIチップの市場、技術、プレイヤーを分析しています。6つの分野(3つの主要地域、エッジとクラウド処理タイプ、4つのデバイスアーキテクチャ(GPU、CPU、ASIC、FPGA)、3つのデバイスパッケージタイプ、4つのアプリケーション分野、9つの業界垂直分野)における10年間(2033年まで)のきめ細かい予測と、最先端AIチップの設計、製造、ATP、運用に関する独自試算を含みます。本レポートは、AIチップの様々な部分に大きなチャンスがあることを明らかにしており、AIチップ市場は2033年までに2500億米ドル以上に成長すると予測しています。
「AIチップ 2023-2033年」が対象とする主なコンテンツ
(詳細は目次のページでご確認ください)
● 全体概要
● 市場予測:
□ 最先端ノードデザイン、製造、組立、テスト、パッケージング、運用コスト試算
□ 後縁ノードに対する先端ノードの製造と運用のコスト比較と分析
□ 包括的AIチップ予測: 多目的チップやクラウドベースのチップを除く包括的調査
□ 詳細予測: 地域(北米、APAC、ヨーロッパ、その他地域)、処理タイプ(エッジ、クラウド)、アーキテクチャ(GPU、CPU、ASIC、FPGA)、パッケージング(SoC、MCM、2.5D+)、アプリケーション(言語、コンピューター・バージョン、予測、その他)、業界特定分野(工業、医療、自動車、小売、メディア・広告、BFSI、消費家電、IT・通信、その他)
● AIハードウェアの技術概要:
□ AIアクセラレータと処理ユニットのイントロダクション
□ AI チップのアーキテクチャとデザインアプローチ
□ ML作業負荷処理用数字表現
□ トランジスタ技術(物理的背景と動向)
□ AIパフォーマンスのベンチマーク比較
● AIチップの展望と近年の製造対象投資:
□ サプライチェーンと有力企業製造能力
□ 米国2022年半導体・科学法、欧州2022年半導体法、中国政府の半導体投資(「中国製造2025」を含む)、韓国政府の半導体投資を含む主要国の資金投入分析
● インタビューを盛り込んだ企業概要
「AIチップ 2023-2033年」は以下の情報を提供します
本レポートは、AIハードウェア、特に機械学習ワークロードの高速化に使用されるチップに関する重要な市場情報を提供します。
最先端AIチップの独自試算
- AIアプリケーションに使用される市場をリードするGPUの仕様に基づいた仮想の3nm GPUのモデルを使用し、GPUの設計、製造、組立、テスト&パッケージングに関連するコストを導き出します。また、最先端ノードと後縁ノードの運用コストも算出し、最先端ノードと後縁ノードの数年にわたる展開の費用対効果を考察します。
市場予測と分析:
- 2023-2033年の市場予測(6分類: 地域、処理タイプ、アーキテクチャ、パッケージング、アプリケーション、業界特定分野)
- 市場予測分析
AIチップを支える技術検証:
- AIデザインと製造の沿革と背景
- さまざまアーキテクチャ概要
- AIチップの一般的機能
- トランジスタ技術の背後にある物理学検証
- トランジスタ技術開発と業界/企業ロードマップの検証
- AIチップ業界で利用されるベンチマーク比較分析
各主要AIチップ製品の包括的市場分析:
- デザインとチップのアーキテクチャ、サプライチェーン、メーカーの製造能力の沿革と動向を含むAIチップの展望検証
- 現在AIチップをデザインする有力企業19社の企業概要(最新・開発中チップ技術に焦点)
- AIチップを開発する最有望のベンチャー企業検証
半導体製造への企業と政府の出資検証:
- 国や地域の半導体製造能力を引き上げるための世界主要国の取り組みの検証と分析
- 世界主要半導体メーカーの検証
The global AI chips market will grow to US$257.6 billion by 2033, with the three largest industry verticals at that time being IT & Telecoms, Banking, Financial Services and Insurance (BFSI), and Consumer Electronics. Artificial Intelligence is transforming the world as we know it; from the success of DeepMind over Go world champion Lee Sedol in 2016, to the robust predictive abilities of OpenAI's ChatGPT, the complexity of AI training algorithms is growing at a startlingly fast pace, where the amount of compute necessary to run newly-developed training algorithms appears to be doubling roughly every four months. In order to keep pace with this growth, hardware for AI applications is needed that is not just scalable - allowing for longevity as new algorithms are introduced, while keeping operational overheads low - but is also able to handle increasingly complex models at a point close to the end-user. A two-pronged approach, to handle AI in the cloud and at the edge, is required to fully realize an effective Internet of Things.
Following a period of dedicated research by expert analysts, IDTechEx has published a report that offers unique insights into the global AI chip technology landscape and corresponding markets. The report contains a comprehensive analysis of 19 players involved with AI chip design, as well as an account of 10 design start-up companies, and the most prominent semiconductor manufacturers globally. This includes a detailed assessment of technology innovations and market dynamics. The market analysis and forecasts focus on total revenue (all-inclusive, excluding multi-purpose, and excluding multi-purpose and cloud-based offerings), with granular forecasts that are disaggregated by geography (Europe, APAC, and North America), processing type (edge and cloud), chip architecture (GPU, CPU, ASIC and FPGA), packaging type (System-on-Chip, Multi-Chip Module, and 2.5D+), application (language, computer vision, predictive, and other), and industry vertical (industrial, healthcare, automotive, retail, media & advertising, BFSI, consumer electronics, IT & telecoms, and other).
In addition, this report contains rigorous calculations pertaining to costs of manufacture, design, assembly, test & packaging, and operation for chips at nodes from 90 nm down to 3 nm, for AI purposes. Forecasts are presented on the design costs and manufacture costs (investment per wafer) as semiconductor manufacturers move to more advanced nodes beyond 3 nm. The report presents an unbiased analysis of primary data gathered via our interviews with key players, and it builds on our expertise in the semiconductor and electronics sectors.
This research delivers valuable insights for:
- Companies that require AI-capable hardware.
- Companies that design/manufacture AI chips and/or AI-capable embedded systems.
- Companies that supply components used in AI-capable embedded systems.
- Companies that invest in AI and/or semiconductor design, manufacture, and packaging.
- Companies that develop other technologies for machine learning workloads.
The rise of intelligent hardware
The notion of designing hardware to fulfil a certain function, particularly if that function is to accelerate certain types of computations by taking control of them away from the main (host) processor is not a new one; the early days of computing saw CPUs (Central Processing Units) paired with mathematical coprocessors, known as Floating-Point Units (FPUs), the purpose of which was to offload complex floating point mathematical operations from the CPU to this special-purpose chip, as the latter could handle computations in a more efficient manner, thereby freeing the CPU up to focus on other things. As markets and technology developed, so too did workloads, and so new pieces of hardware were needed to handle these workloads. A particularly noteworthy example of one of these specialized workloads is the production of computer graphics, where the accelerator in question has become something of a household name: the Graphics Processing Unit (GPU).
Just as computer graphics required a different type of chip architecture, so the emergence of machine learning has brought about a demand for another type of accelerator, one that is capable of efficiently handling machine learning workloads. This report details the differences between CPU, GPU and Field Programmable Gate Array (FPGA) architectures, and their relative effectiveness with handling machine learning workloads. Application-specific Integrated Circuits (ASICs) can be effectively designed to handle specific workloads, with the architectures of several of the world's leading designers of ASICs for AI being analyzed in this report. The need for chips capable of handling ML workloads will only increase as the benefits for consumers (increased functionality in consumer electronics, more accurate image classification and object detection in security cameras, and low latency, high-precision inference in autonomous vehicles, for example) is realized, which is reflected in the forecast compound annual growth rate (CAGR) of 24.4% for AI chips (including those that are used for other purposes in addition to handling ML workloads, as well as chips accessible through a cloud service) between the years 2023 and 2033.
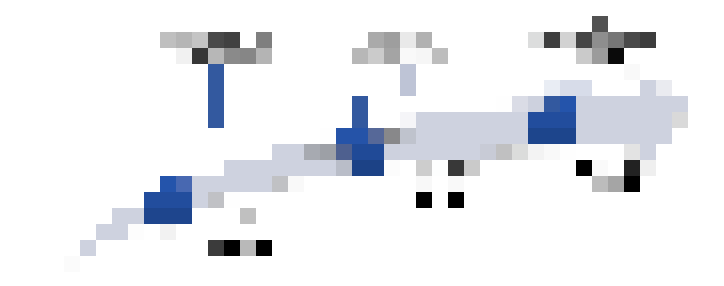
Compound Annual Growth Rates for each of the three main forecasts in this report, between the years 2023 and 2033. Source: IDTechEx
AI is on the global agenda
AI's capabilities in natural language processing (understanding of textual data, not just from a linguistic perspective but also a contextual one), speech recognition (being able to decipher a spoken language and convert it to text in the same language, or convert to another language), recommendation (being able to send personalized adverts/suggestions to consumers based on their interactions with service items), reinforcement learning (being able to make predictions based on observations/exploration, such as is used when training agents to play a game), object detection, and image classification (being able to distinguish objects from an environment, and decide on what that object is) are so significant to the efficacy of certain products (such as autonomous vehicles and industrial robots) and to models of national governance, that the development of AI hardware and software has motivated national and regional funding initiatives across the globe. As AI-capable processors and accelerators are dependent on semiconductor manufacturers, with those capable of producing the more advanced nodes necessary for chips employed within data centres located in the Asia-Pacific region (particularly Taiwan and South Korea), the ability to manufacture AI chips is dependent on the possible supply from a select few companies (for edge devices, it is not as necessary to employ leading-edge node technology, given that these chips are typically used for low-power inference. However, the fact remains that the global supply chain is heavily indebted to a specific geographic region).
The risk of relying on the manufacturing capabilities of companies concentrated in a specific geographic region was realized in 2020, when a number of complementing factors (such as the COVID-19 pandemic, the rise of data mining, a Taiwanese drought, fabrication facility fire outbreaks, and neon procurement difficulties) led to a global chip shortage, where demand for semiconductor chips exceeded supply. Since then, the largest stakeholders in the semiconductor value chain (the US, the EU, South Korea, Taiwan, Japan, and China) have sought to reduce their exposure to a manufacturing deficit, should another set of circumstances arise that results in an even more exacerbated chip shortage. National and regional government initiatives have been put in place to incentivize semiconductor manufacturing companies to expand operations or build new facilities. These government initiatives are discussed in the report, where the funding is broken down and the reasons for these initiatives and what they mean for other stakeholders (such as the restrictions imposed on China by the US, and how China can build a national semiconductor supply chain around these restrictions) is detailed. In addition, the private investments announced for semiconductor manufacture since 2021 are outlined, along with current company semiconductor manufacture capabilities, particularly in relation to AI.
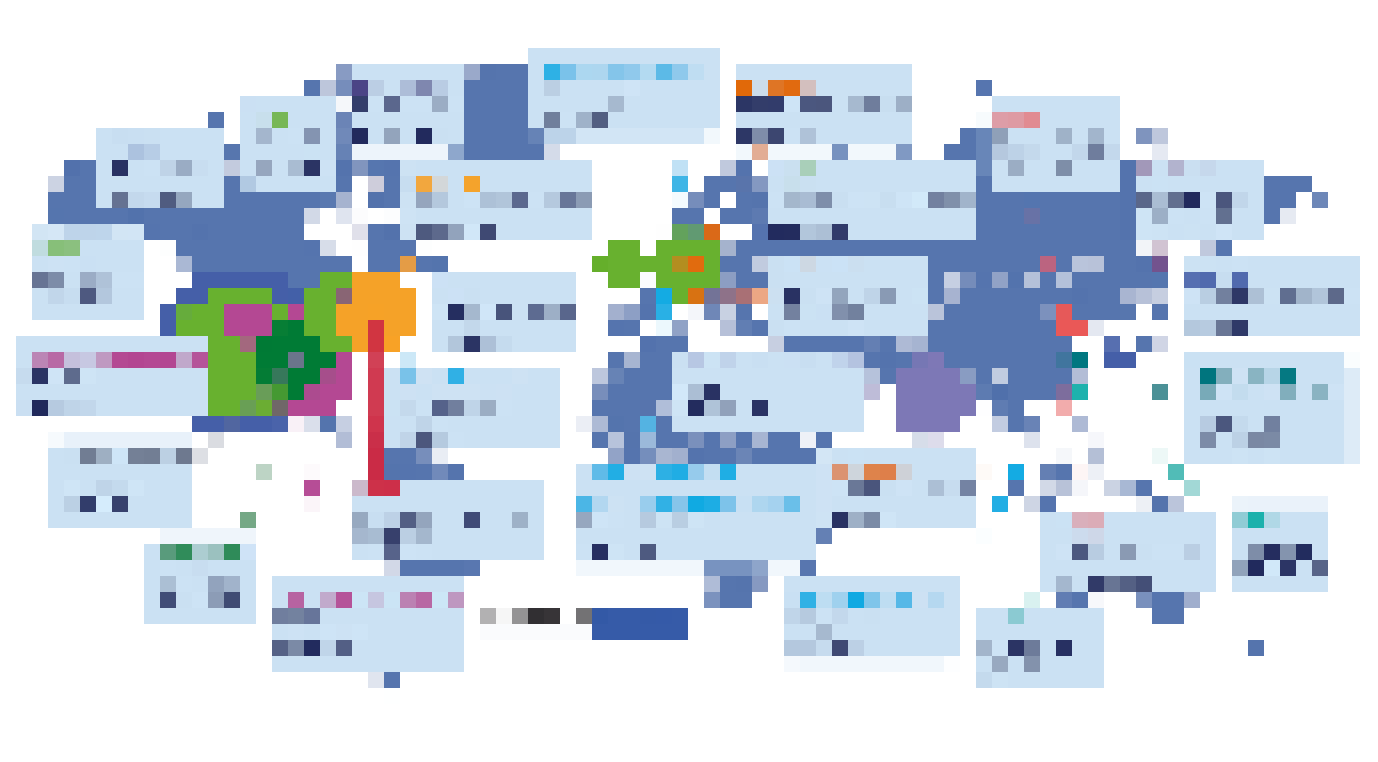
Shown here are the proposed and confirmed investments into semiconductor facilities by manufacturers since 2021. Where currencies have been listed in anything but US$, these have been converted to US$ as of publication date. Source: IDTechEx
The cost of progress
Machine learning is the process by which computer programs utilize data to make predictions based on a model, and then optimize the model to better fit with the data provided, by adjusting the weightings used. Computation therefore involves two steps: Training, and Inference. The first stage of implementing an AI algorithm is the training stage, where data is fed into the model and the model adjust its weights until it fits appropriately with the provided data. The second stage is the inference stage, where the trained AI algorithm is executed, and new data (that was not provided in the training stage) is classified in a manner consist with the acquired data. Of the two stages, the training stage is more computationally intense, given that this stage involves performing the same computation millions of times (the training for some leading AI algorithms can take days to complete). This then poses the question: how much does it cost to train AI algorithms?
In an effort to quantify this, IDTechEx has rigorously calculated the design, manufacture, assembly, test & packaging, and operational costs of AI chips from 90 nm down to 3 nm. By considering that a 3 nm chip with a given transistor density will have a smaller area than a more mature node chip with the same transistor density, the cost of deploying a leading-edge chip for a given AI algorithm can be compared with a trailing-edge chip capable of a similar performance for the same algorithm. For example, should a 3 nm chip with a given area and transistor density be used continuously for five years, the cost incurred will be 45.4X less than the cost incurred by running a 90 nm chip with the same transistor density continuously for five years, based on the model of a 3 nm chip that we employ. This includes the initial production costs of the respective chips, and can then be used to determine whether it is worthwhile to upgrade from a more mature node chip to a more advanced node chip, depending on how long the chip is to be in service for.
The costs associated with producing and operating a chip at each of the given nodes over the course of 5 years, based on our model of a 3 nm chip used for AI purposes. Source: IDTechEx
Market developments and roadmaps
IDTechEx's model of the global AI chips market considers architectural trends, developments in packaging, the dispersion/concentration of funding and investments, historical financial data, and geographically-localized ecosystems to give an accurate representation of the evolving market value over the next ten years.